How Infectious Disease Surveillance works in India?
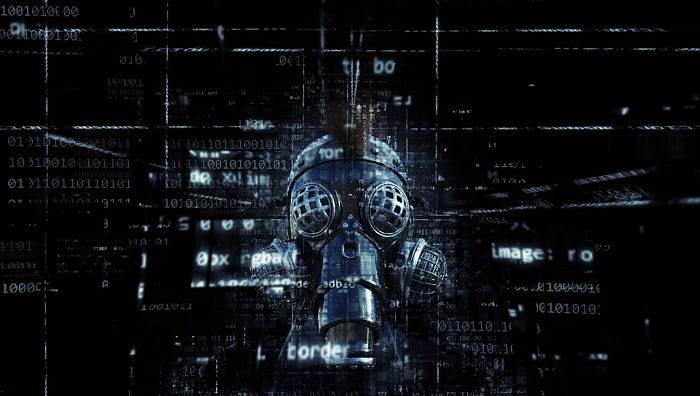
With novel emerging cases of disease like the “mysterious” disease in Eluru, a town in the Indian State of Andhra Pradesh, a public health surveillance of infectious diseases is more important than ever before.
But why is this surveillance needed?
· Describing the burden and epidemiology of disease
· In monitoring the trend to control, eliminate, and eradicate diseases by guiding public health program interventions, policies, and strategies
· Documenting the impact of an intervention or progress towards a specified public health targets/goals
Note: Data depicted below are not actual data and is only for education purposes.
Basic components of surveillance include:
a) Collection, b) Analysis, c) Dissemination and d) Response
Let us simplify how Collection and Analysis are done using a sample dataset.
Step 1: Collection of Data
I have collected the data taking reference from Integrated Disease Surveillance Program
A look at our initial 10 data:

Count: Total occurrences of each disease
Rate: Count of disease per population
Step 2: Analysis
Generalizing to get each Disease count per year across the locations, we get:-

Let us visualize this data and analyze a trend.

Trends for some of the most infectious diseases:
a) Dengue had an abrupt fall in 2013-2014, why?
b) Chickenpox was almost stagnant throughout 2001–2014, even with increase in population, why?
c) Viral Fever had the highest increase rate among the others.
Similarly let us also visualize to get Density of Diseases in each location per year, we get:

Among the States, Chhattisgarh had the highest Disease density. And density has sharply increased from 2009. In comparison for other states, disease density has almost remained constant.
Let us also visualize the data for the total Disease Count per location.

Result: Andaman & Nicobar [AN] had the highest count of Acute Diarrhoeal Disease, followed by Delhi. AN also had the highest count of Food Poisoning, followed by Arunachal Pradesh.
Now coming to the magic section, let us use Machine Learning to predict the future values. I have used Linear-Regression analysis for the statistical purpose.
For our purpose, I am using this model to predict the future values of Acute Diarrhoeal Disease in Andaman & Nicobar. I have taken “Year” and “Population” as independent variable and “Count” as dependent variable.
[Note: Count of a disease have several more other factors. For simplification of our analysis, I have considered only these variables]
After fitting the variables on the model, let us predict the values on the same variables and evaluate the performance of linear-regression model using Co-efficient of Determination: R2-score.

Result: In our case, R2-score came out to be 72% which means that it can be referred that 72% of the changeability of the dependent output attribute can be explained by the model while the remaining 28 % of the variability is still unaccounted for.
Takeaway
As widely said “Prevention is better than cure”, prediction of diseases and epidemic outbreak would lead to an early prevention of an occurrence of a disease. Medical facilities need to be advanced so that better decisions for patient diagnosis and treatment options can be made.
Using the sample dataset, we got an overall idea of:
a) Visualizing the data provided a pattern which will lead to an in-depth analysis. Eg: Out of top Infectious Disease in India from 2001–2014, Dengue had a sharp downfall in 2013–2014. The probable answers could be:
- Strengthening of Health system
- Improvement of Health Information and Research System
- Introduction of bacteria-infected mosquitoes (Highly-unlikely!!)
b) Using Machine Learning, we can predict for any outbreak of diseases. Eg: In our case, we found that Acute Diarrhoeal Disease in Andaman & Nicobar will have a sharp increase of count in year 2014 - 2016.
Disease prediction has the potential to benefit stakeholders such as National Health Programs and the government. It can identify patients at risk of disease or health conditions. Clinicians can then take appropriate measures to avoid or minimize the risk and in turn, improve quality of care and avoid potential hospital admissions.
Machine learning in healthcare aids the humans to process huge and complex medical datasets and then analyze them into clinical insights. This then can further be used by physicians in providing medical care. Hence machine learning when implemented in healthcare can leads to increased patient satisfaction. In our analysis, we tried to implement functionalities of machine learning in healthcare in a single system. Instead of diagnosis, when a disease prediction is implemented using certain machine learning predictive algorithms then healthcare can be made smart.
hope you enjoy reading this :)